Overview
Estimated Reading Time
Plan on around 90 - 120 minutes for this preparation reading, which consists of textbook reading.
There are also some optional online materials you may want to review.
The objective of this module is to provide a real-world scenario in which you can practice the following data science / machine learning skills:
- Decision Trees and the ID3 Algorithm
- Testing a machine learning model
- Evaluating how well a model makes categorical predictions
Preparation Reading
Reading the Text
As you read the chapters in the textbook, you may find it useful to start with the chapter summary at the end of the chapter.
This summary provides an overview of the key concepts presented in the chapter, and shows how each is connected together.
Sensitivity and Specificity
In section 8.4.2.1 (Confusion Matrix-based Performance Measures), you'll see the terms "True Positive Rate" and "True Negative Rate" (among others).
It's more common to refer to "True Positive Rate" as Sensitivity.
It is also more common to refer to "True Negative Rate" as Specificity.
The text mentions this in a footnote, but they are such common terms, we want to make sure you don't miss this definition.
Complete the following preparation reading:
-
Read Chapter 4 until section 4.4 in the textbook, which describes Decision Trees and the ID3 algorithm.
-
Read the following sections from chapter 8 which each describe a specific model performance measure:
- 8.4.2.1 - Confusion Matrix-based Performance Measures
- 8.4.2.2 - Precision, Recall, and $F_1$ Measure.
- 8.4.3 - Prediction Scores
- 8.4.3.1 - Receiver Operating Characteristic Curves (ROC Curves)
Decision Trees
You can find documentation on how to use decision trees with sci-kit learn on these pages:
Extra Help
Below you'll find some optional videos and other resources that help supplement the reading.
You should absolutely still do the reading above. One technique would be to read the text, paying particular attention to new concepts (usually written in bold), then research those concepts using videos or other articles until you're confident you understand them. Afterwards, circle back to the text to pick up extra details you might have missed the first time.
Learning Complex Technical Information
Reading technical information can be difficult and is an acquired skill that you absolutely should develop if you're planning to work in data science. New research papers and algorithms are released constantly in this field that require you to parse through information and formulas.
This helps you to not only understand how the algorithm works, but which types of problems the algorithm would and would not not be suited for.
However, sometimes it's nice to have a different perspective. Some people learn better visually, through videos, interactively, or by example.
In some cases, a superficial understanding of an algorithm and its parameters may be good enough for what you need to do. But you'll always benefit from a deeper understanding of how the tools and algorithms you're using actually work, and the reasons they behave better in some situations than others.
Decision Trees
- Here's a cool interactive demo of decision trees.
- This follow-up demo shows the bias-variance trade-off in decision tree models.
Shannon's Entropy Model
Image from The Intuition behind Shannon’s Entropy
-
This video has a nice visual way of explaining the relationship between entropy, questions, and information.
-
The Intuition behind Shannon’s Entropy has a nice discussion of the reason behind the log transform part of the entropy formula (equation 4.1 in the text).
-
Why take the log of a continuous target variable has another nice explanation of why we often care about the log transform of a feature, something that's going to come up quite often.
Model Evaluation
- ROC and AUC, Clearly Explained! is a video visually describing how to build and use an ROC curve. (Don't let the corny intro music dissuade you, it's a pretty good video)
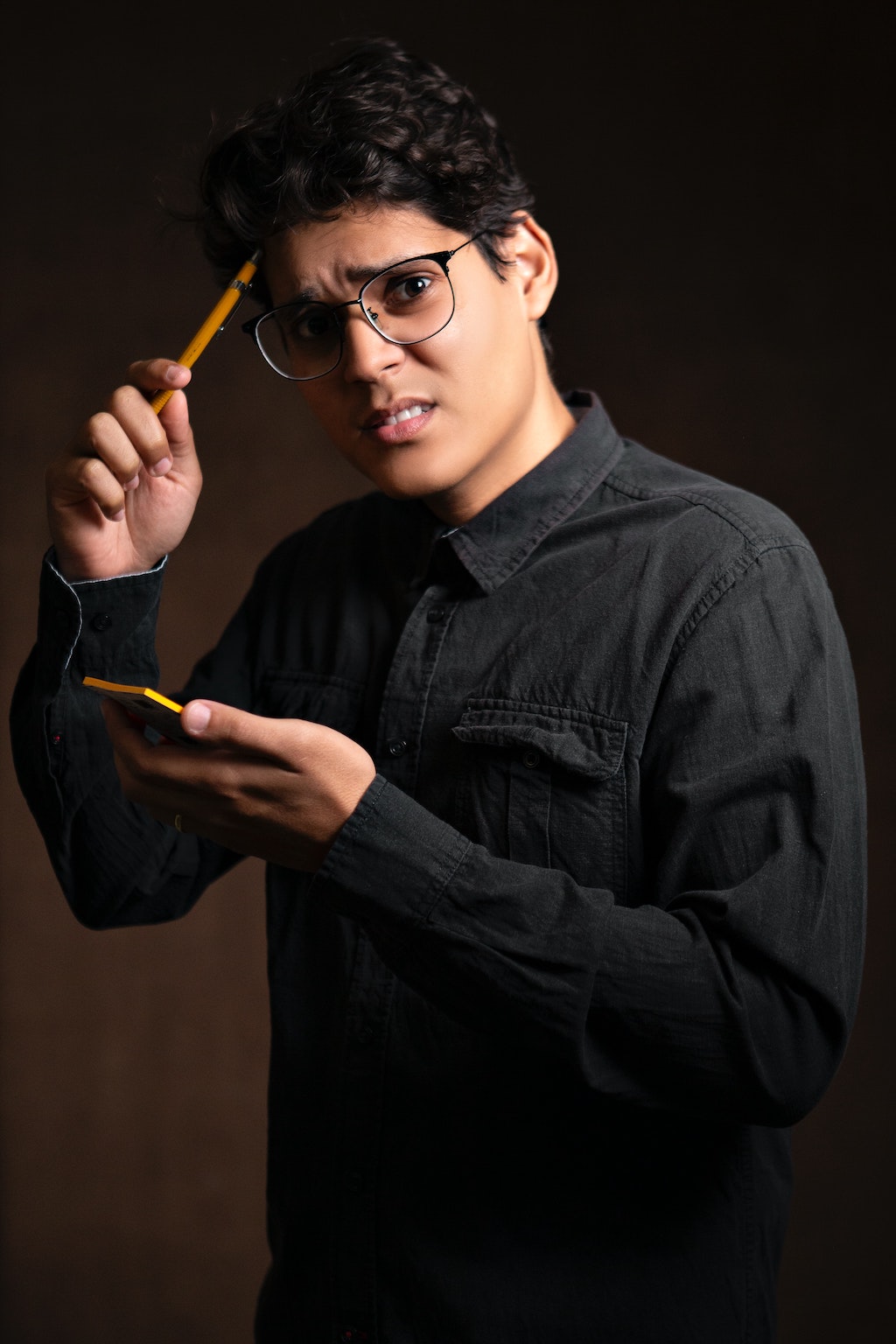
Johnny, the Data Science Intern, drops by your hotel room around midnight:
Okay, just one last thing, if you need any more help at all, I put together this collection of Google Colab notebooks that might be useful.